Spoof? You can’t handle the Spoof
Markets have been stunned recently by regulators hitting high-profile organizations, including tier one investment banks and trading platforms, with significant fines for cases involving ‘spoofing’.
by Steve Wilcockson, Product Marketing, Data Science, KX
Spoofing is a form of market manipulation where a trader places a large series of orders to buy or sell a financial asset, such as a stock, bond, or futures contract, with no intention of executing them. With increasing varieties of interconnected asset classes being traded, organizations must be more alert across all of their markets or risk severe sanctions.
For example, in one case, a trader took advantage of the close correlation between U.S. Treasury securities and U.S. Treasury futures contracts and engaged in cross-market manipulation by placing spoof orders in the futures market to profit in the cash market. This resulted in a $35million fine! Or take the case of precious-metals traders who consistently manipulated the gold and silver market over seven years and lied about their conduct to regulators who investigated them. Penalties are in the order of a billion USD.
A revolution in detection
Such cases represent a clear failure to prevent instances of market abuse, and we might ask how that is possible given recent investments in detection systems designed to help protect organizations from such activity.
Technology on its own is not a solution. Personal ethics are, and always will be, an issue. However, technical evolution in how spoofing is conducted must be countered with a revolution in spoof detection. Traditional spoofing operates where false, but manipulative, orders are placed on the same asset where the unlawful profits may get realized. Traditional systems may capture such instances well. However, systems can and have failed in cases of more furtive manipulation, such as realizing the profit on a derivative by placing the spoofing orders on an underlying asset, not the derivative contract itself.
Successful future-proofed technologies must look for correlations across assets, business units, and markets. But more monitoring means more data and compute overhead, as well as team and workflow challenges.
When monitoring so many more data combinations, static detection systems face challenges. They need to be sufficiently agile and dynamic to handle greater data dimensionality. Robust statistics, machine learning, and behavioural analytics can help quickly synthesize data, provide early indicators of suspicious activities, and quickly eliminate false positives, but more is needed. Delivering rigorous historical event analysis and real-time insights, detection systems and their owners need dimension-busting algorithms that can work with ever-increasing volumes and complexities of data at speed.
Scalable analytics
Detection technologies must adapt to evolving market needs: new data types, the sheer volume of data, and constant updates over time. Time-series data – collections of data, often from different sources and types, organized through time – is the most efficient base unit, enabling ready processing to seek correlations, anomalies, and patterns. For example, when looking for spoofing and “layering” specifically, internal order/quote actions and trades are compared to market quotes, not just top of the book but also in their depth and consolidated trades. This helps determine if deceptive orders and cancellations that formed part of the strategy were marketable (i.e., likely to execute) at the time of the transaction. This can consist of hundreds of millions of records or more. The North American futures industry, for instance, generates over 100 billion order messages each day, and the securities markets billions more!
Choosing the right haystack
Another challenge is finding meaning in the masses of data. In plain terms, when looking for a needle in a haystack, select the right haystack to start with, and then minimize the disruption to finding the needle. In such cases, machine learning can deliver more efficacy over such high-dimensional data than rules-based solutions. Yet rightly or wrongly, and for reasons of regulatory compliance and governance around explainability and reproducibility, machine learning models tend to augment easier-to-validate rules-based processes.
However, machine learning techniques can compute over as many axes as there are useful features, easily. One popular method deployed across many industries and applications – from police surveillance to cybersecurity, from search engine recommendations to predictive healthcare and financial surveillance – is a Support Vector Machine (SVM). This is a great algorithm to identify and score features – measurable pieces of data – such as colours and distances on an image, or, in the financial world, trade characteristics and trading patterns including fraudulent features across different data sets.
Many other algorithms and tests apply in addition to SVMs. Whatever the model approach – clustering or regression, linear or nonlinear, machine or deep learning algorithms – their parameterization is invaluable in financial surveillance. For spoofing, they can navigate well the frontiers and layers of normal and abnormal market activities, and assess balanced and unbalanced markets, where liquidity might be illusory or volume artificial.
Conclusion
Spoofing is hard to detect. Its very existence relies on trades likely not being executed, increasingly across different markets and assets. As the examples have shown, regulators have the teeth to find and punish such market-abusing spoofers, so regulated entities need to ensure they have the tools to find them too. Personal ethics will forever challenge financial organizations and regulators, but dynamic, flexible, fast technologies navigating highly complex data sets can future-proof organizations, adding agility and scalability to their fraud detection, crime, and AML stacks.
IBSi News
Get the IBSi FinTech Journal India Edition
- Insightful Financial Technology News Analysis
- Leadership Interviews from the Indian FinTech Ecosystem
- Expert Perspectives from the Executive Team
- Snapshots of Industry Deals, Events & Insights
- An India FinTech Case Study
- Monthly issues of the iconic global IBSi FinTech Journal
- Attend a webinar hosted by the magazine once during your subscription period
₹200 ₹99*/month
* Discounted Offer for a Limited Period on a 12-month Subscription
IBSi FinTech Journal
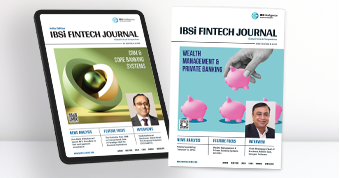
- Most trusted FinTech journal since 1991
- Digital monthly issue
- 60+ pages of research, analysis, interviews, opinions, and rankings
- Global coverage
Other Related Blogs
April 10, 2025
Is “OS Approach” the answer to enhancing Legacy Systems & Process Automation in Private Markets?
Read MoreJune 06, 2022
Sustaining the future of trade: How FinTechs are making global trade more inclusive
Read MoreRelated Reports
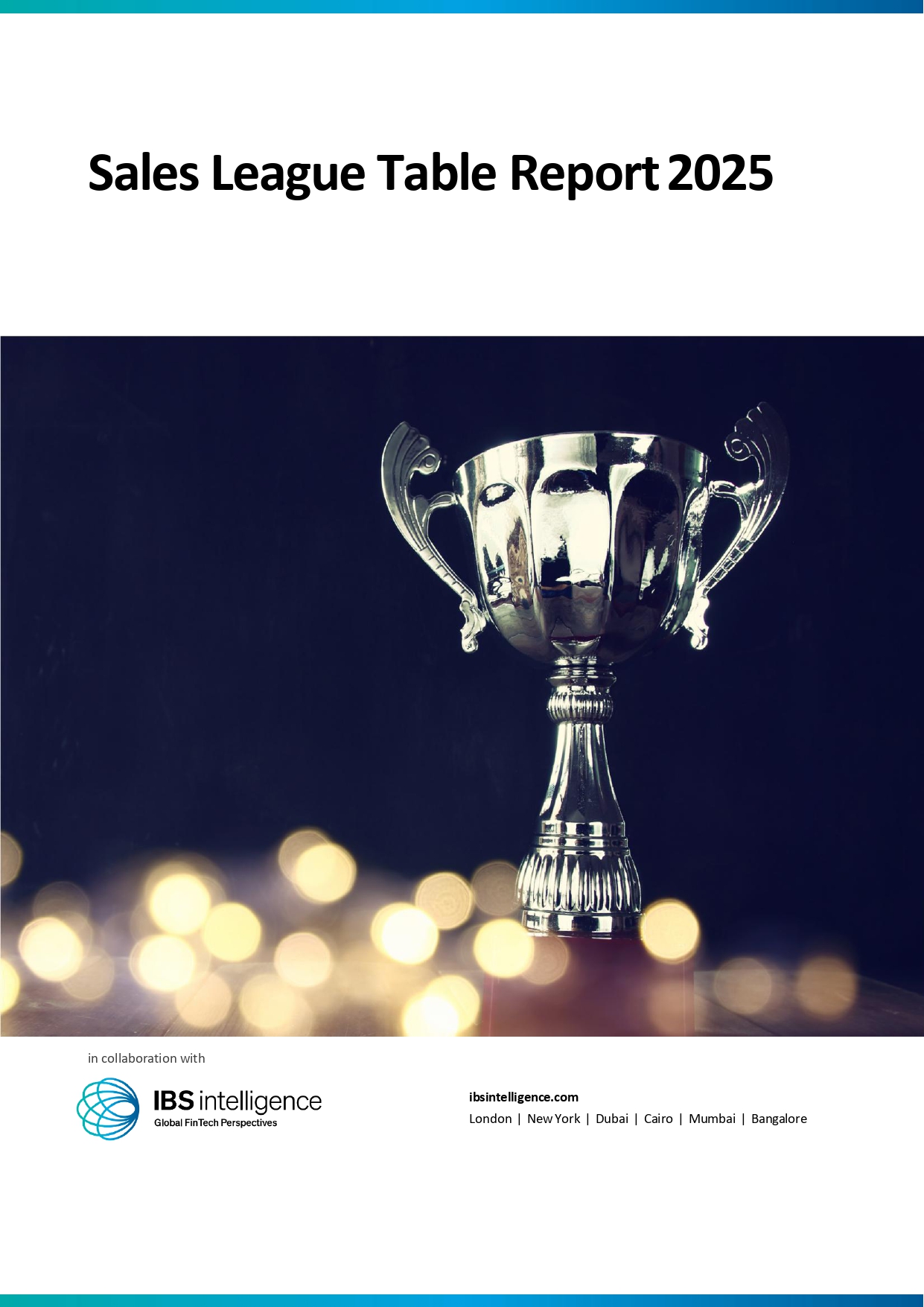
Sales League Table Report 2025
Know More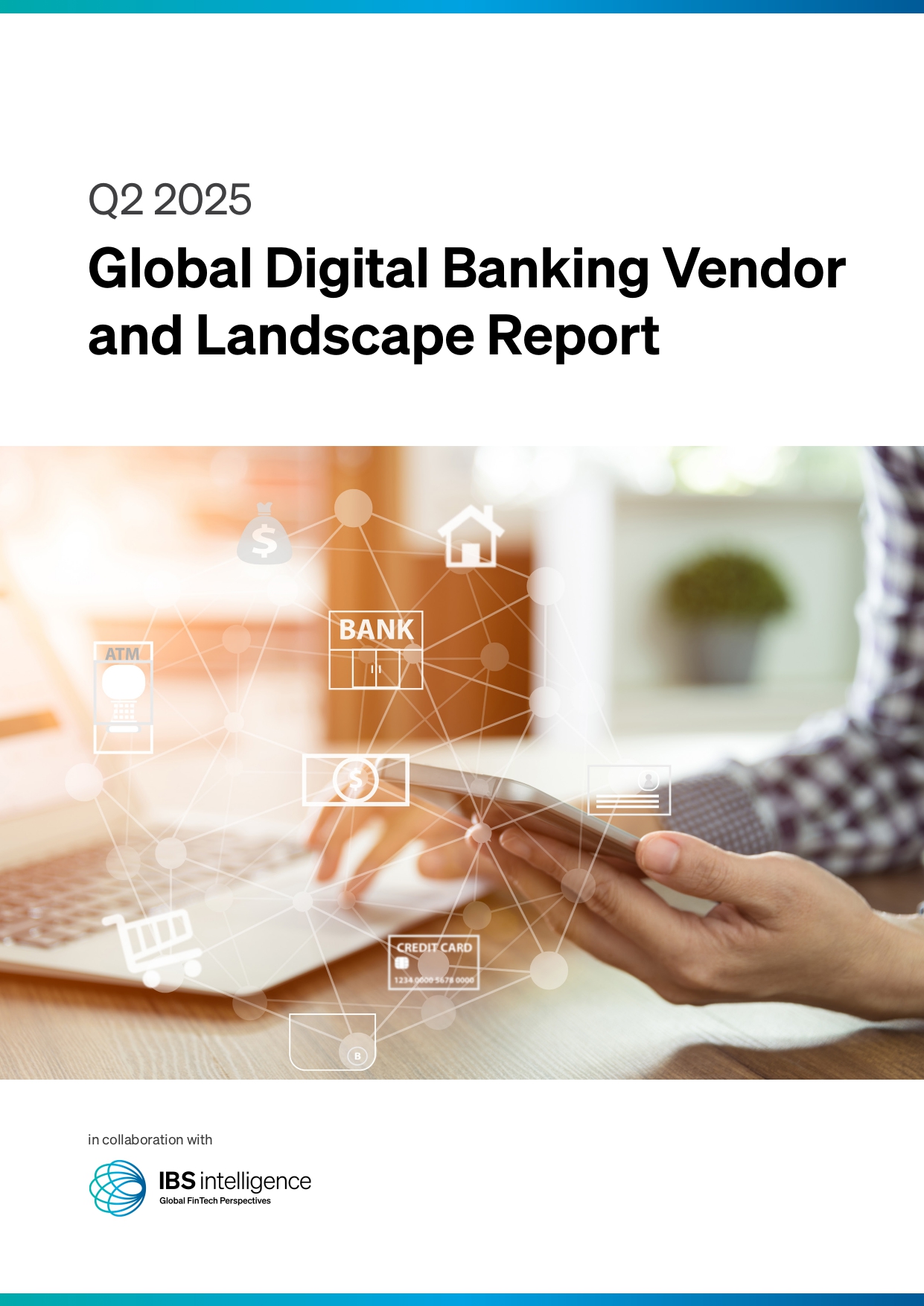
Global Digital Banking Vendor & Landscape Report Q2 2025
Know More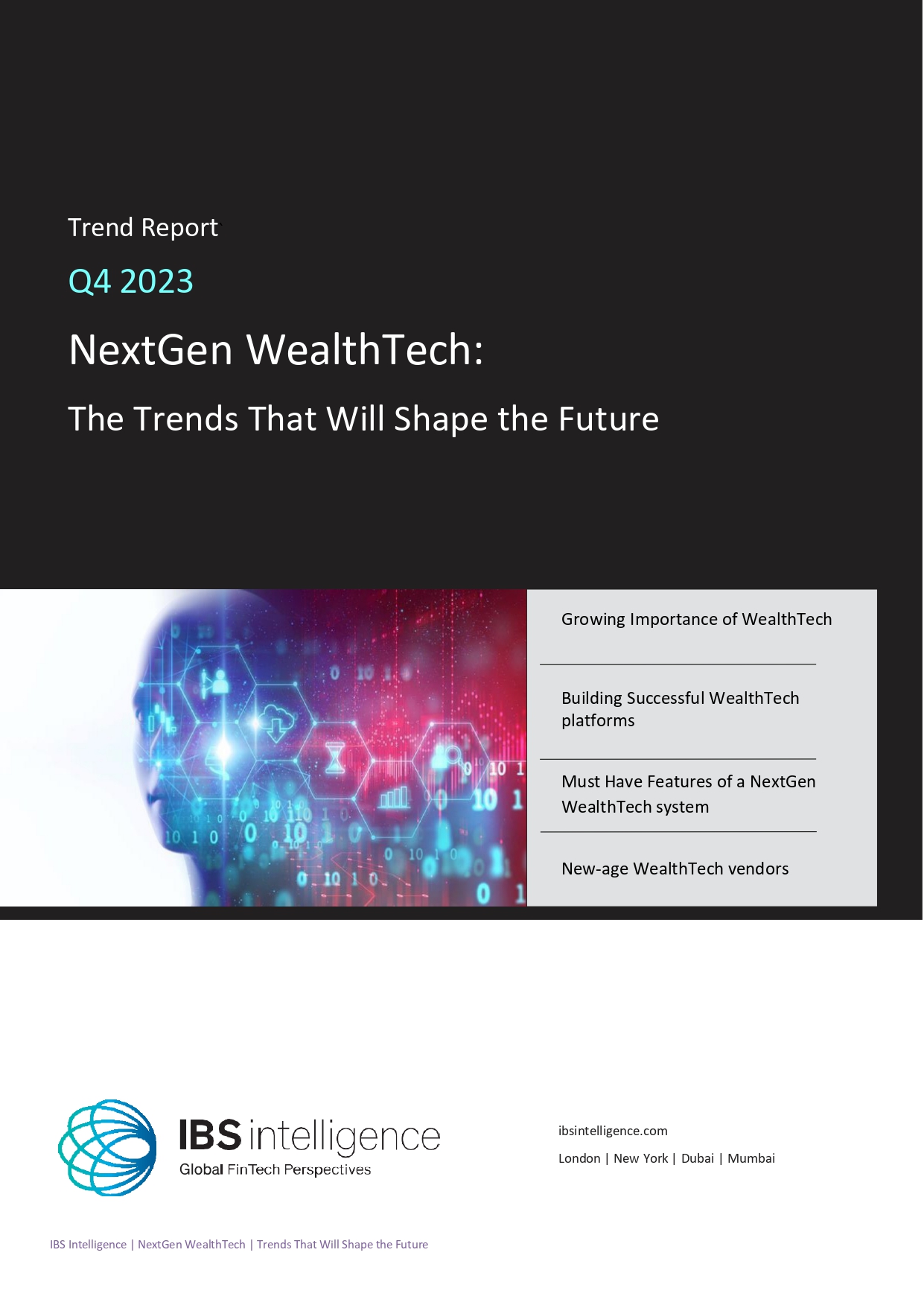
NextGen WealthTech: The Trends To Shape The Future Q4 2023
Know More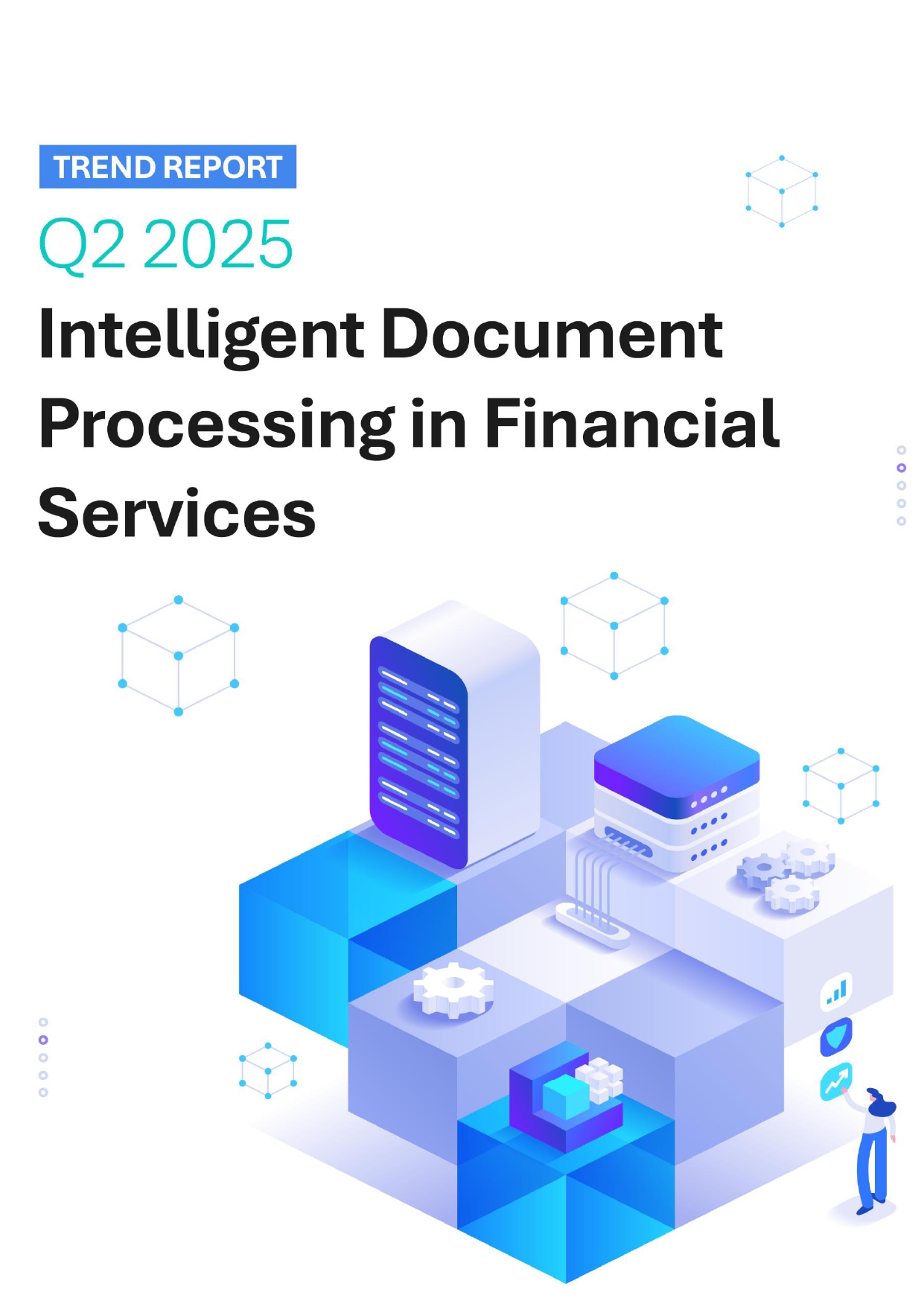
Intelligent Document Processing in Financial Services Q2 2025
Know More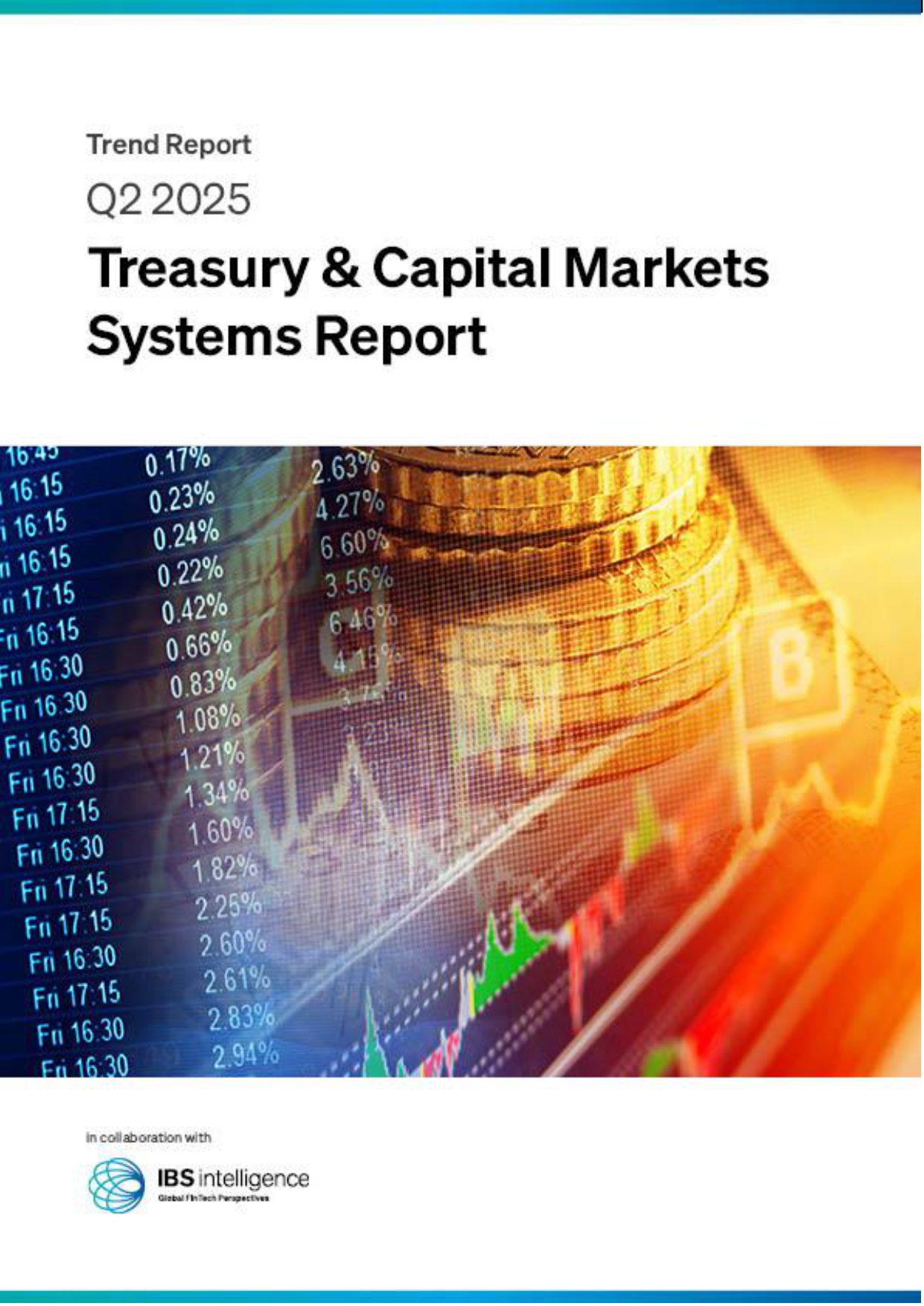