Big data analytics set to transform banking with projected $745bn market by 2030
By Puja Sharma
The Big Data Analytics in the Banking market, a key player in addressing the industry’s challenges, is propelled by the increasing demand for personalised customer experiences and risk management. It tackles hurdles such as data security, regulatory compliance, and integration with legacy systems. The market’s growth is fueled by the increasing digital transformation and the need for real-time insights, while the complexity of data management and talent shortages remain significant obstacles.
The Global Big Data Analytics in Banking Market is projected to grow at a CAGR of 13.5% from 2024 to 2030, according to a report published by Verified Market Reports. The report reveals that the market was valued at $307.52 Billion in 2023 and is expected to reach $745.16 Billion by the end of the forecast period.
Big Data Analytics in Banking Market Overview
Big Data Analytics has revolutionised the banking sector by enabling institutions to process vast amounts of data in real time, providing valuable insights that drive decision-making. The integration of Big Data technologies in banking has shifted the industry from traditional methods to data-driven strategies, improving everything from customer experience to risk management.
Banks collect and analyse data from various sources, including transactions, social media, and customer feedback, to gain a comprehensive understanding of market trends and customer behaviours. This shift has allowed for more personalised services and better predictive modelling, which are essential in today’s competitive financial landscape.
Market Drivers and Growth Factors
The rapid growth of digital banking and the increasing volume of unstructured data are key drivers of the Big Data Analytics market in banking. Customers now demand seamless digital experiences, pushing banks to adopt advanced analytics tools to meet these expectations.
Furthermore, the rise in cyber threats and the need for robust security measures have made Big Data Analytics indispensable for fraud detection and compliance. The ability to analyse large datasets helps banks to identify patterns that can predict fraudulent activities, thus protecting both the institution and its customers.
Additionally, regulatory requirements demand more sophisticated data management and reporting, further fueling the adoption of Big Data Analytics in the banking sector.
Key Technologies and Tools
Various technologies and tools, such as Hadoop, Apache Spark, and machine learning algorithms, form the backbone of big data analytics in banking. These technologies allow banks to process and analyse large volumes of data efficiently.
Hadoop provides a distributed storage system that enables banks to manage big datasets across different nodes, while Apache Spark enhances the speed of data processing.
Machine learning, on the other hand, plays a crucial role in predictive analytics, helping banks forecast market trends and customer needs. The integration of these technologies not only improves data processing capabilities but also supports real-time analytics, which is critical in a fast-paced banking environment.
Applications in Customer Relationship Management
Big Data Analytics has significantly enhanced Customer Relationship Management (CRM) in banking. By analysing customer data, banks can offer personalised services that meet the unique needs of each client. This includes tailored product offerings, customised marketing campaigns, and better customer support.
Big Data allows banks to segment their customers based on various factors, such as spending habits, preferences, and financial goals. This segmentation helps create targeted strategies that improve customer satisfaction and loyalty. Moreover, sentiment analysis through social media data enables banks to gauge customer opinions and respond proactively to concerns, thereby enhancing the overall customer experience.
Challenges and Risks
Despite its benefits, the implementation of Big Data Analytics in banking comes with several challenges. Data privacy and security are significant concerns as banks deal with sensitive customer information that must be protected from breaches. The complexity of Big Data tools also requires significant investment in technology and skilled personnel, which can be a barrier for smaller institutions.
Additionally, there is the challenge of integrating Big Data Analytics with existing systems, which may not be designed to handle such large volumes of data. Banks must also navigate the regulatory landscape, ensuring that their data practices comply with laws such as GDPR, which adds another layer of complexity to Big Data initiatives.
Future Trends and Opportunities
The future of Big Data Analytics in banking is promising, with advancements in artificial intelligence (AI) and machine learning set to further enhance the capabilities of banks. AI-driven analytics will enable more accurate predictive models, allowing banks to anticipate market changes and customer needs with greater precision. The use of blockchain technology for secure data sharing is another emerging trend that could revolutionise data management in the banking sector.
Additionally, the continued growth of mobile banking will generate even more data, providing banks with richer datasets to analyse. As these technologies evolve, banks that effectively leverage Big Data Analytics will be well-positioned to gain a competitive edge in the market.
IBSi FinTech Journal
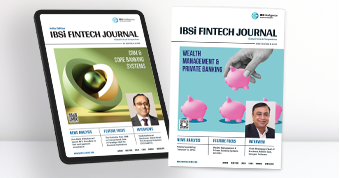
- Most trusted FinTech journal since 1991
- Digital monthly issue
- 60+ pages of research, analysis, interviews, opinions, and rankings
- Global coverage
Other Related News
Related Reports
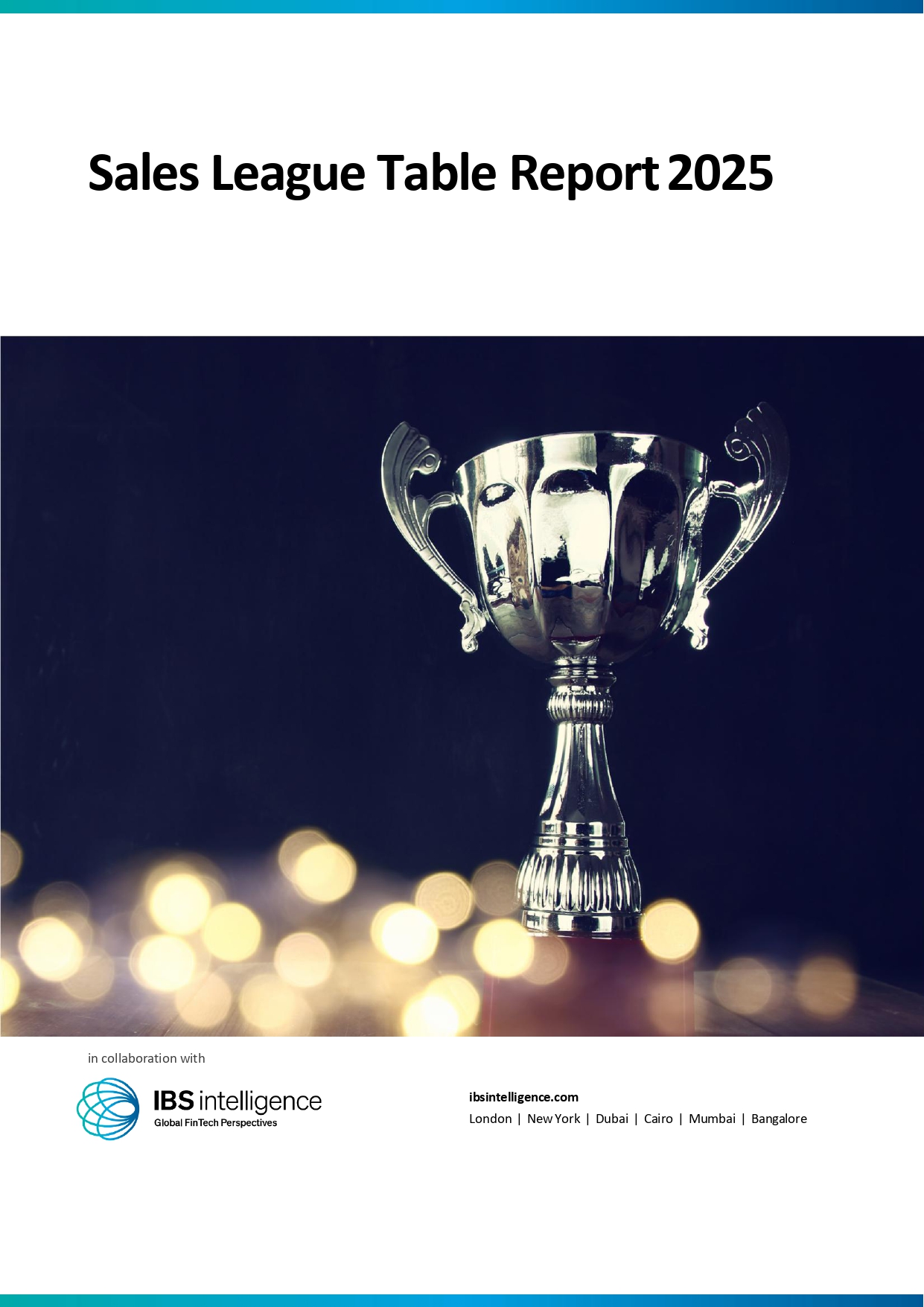
Sales League Table Report 2025
Know More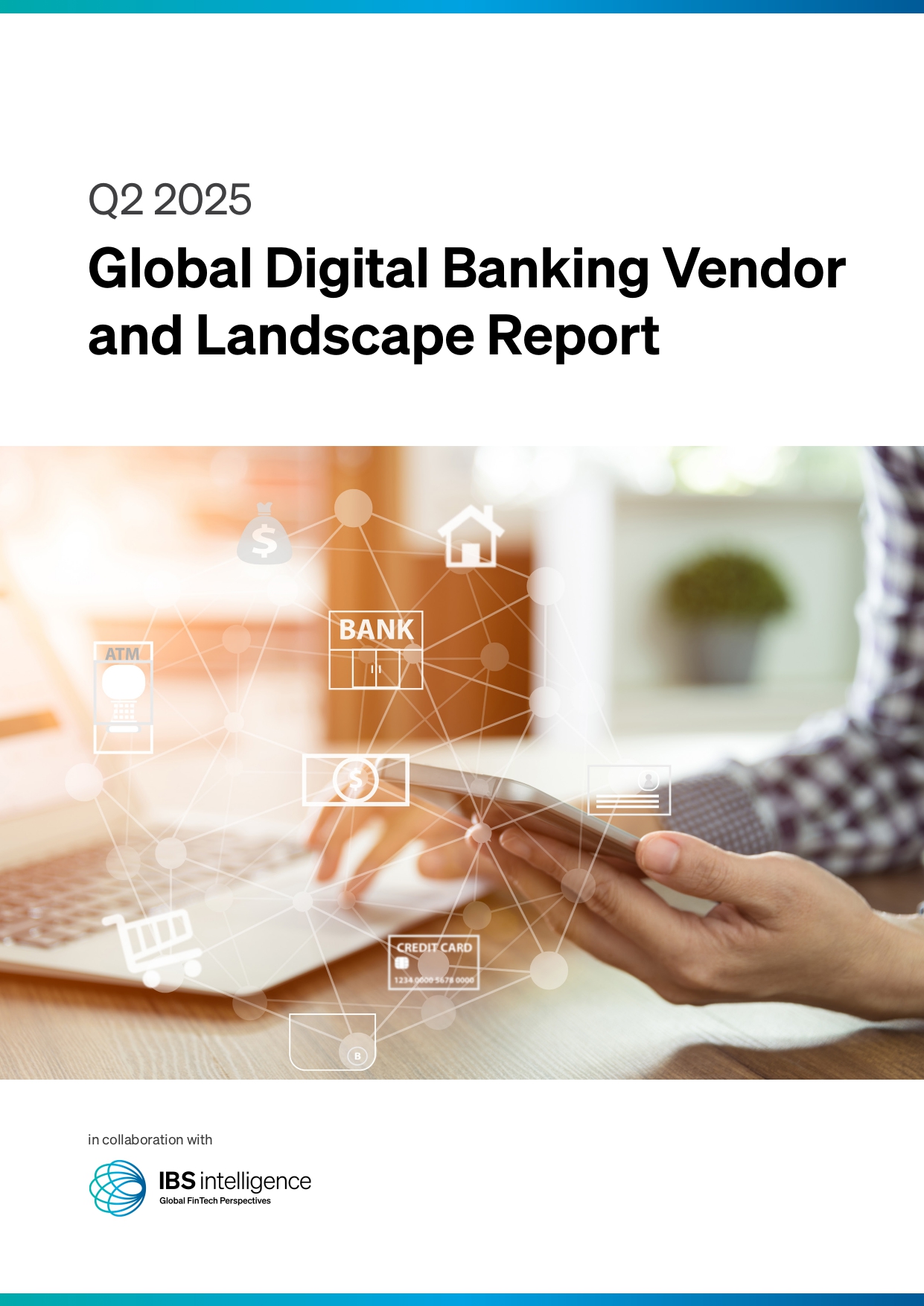
Global Digital Banking Vendor & Landscape Report Q2 2025
Know More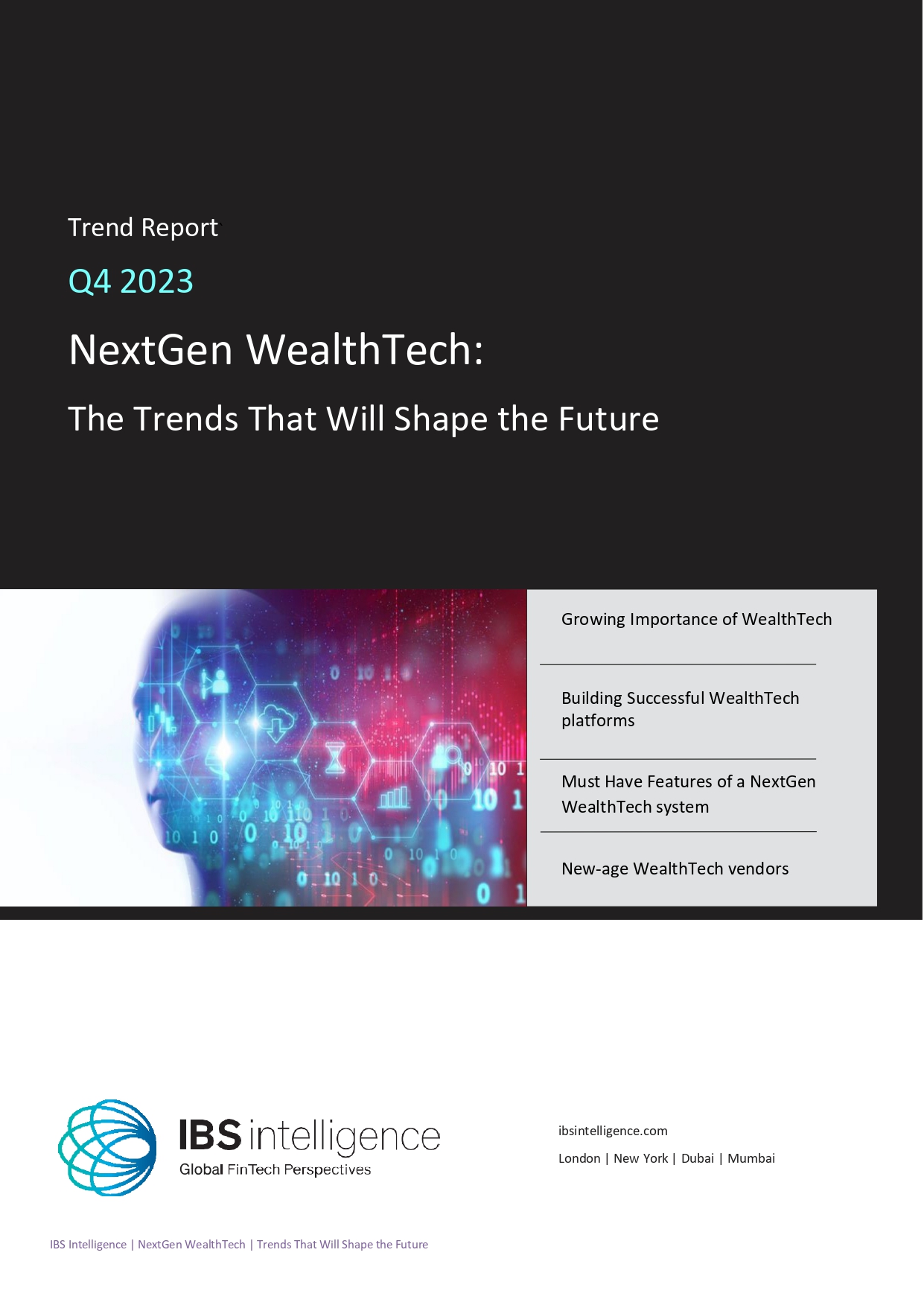
NextGen WealthTech: The Trends To Shape The Future Q4 2023
Know More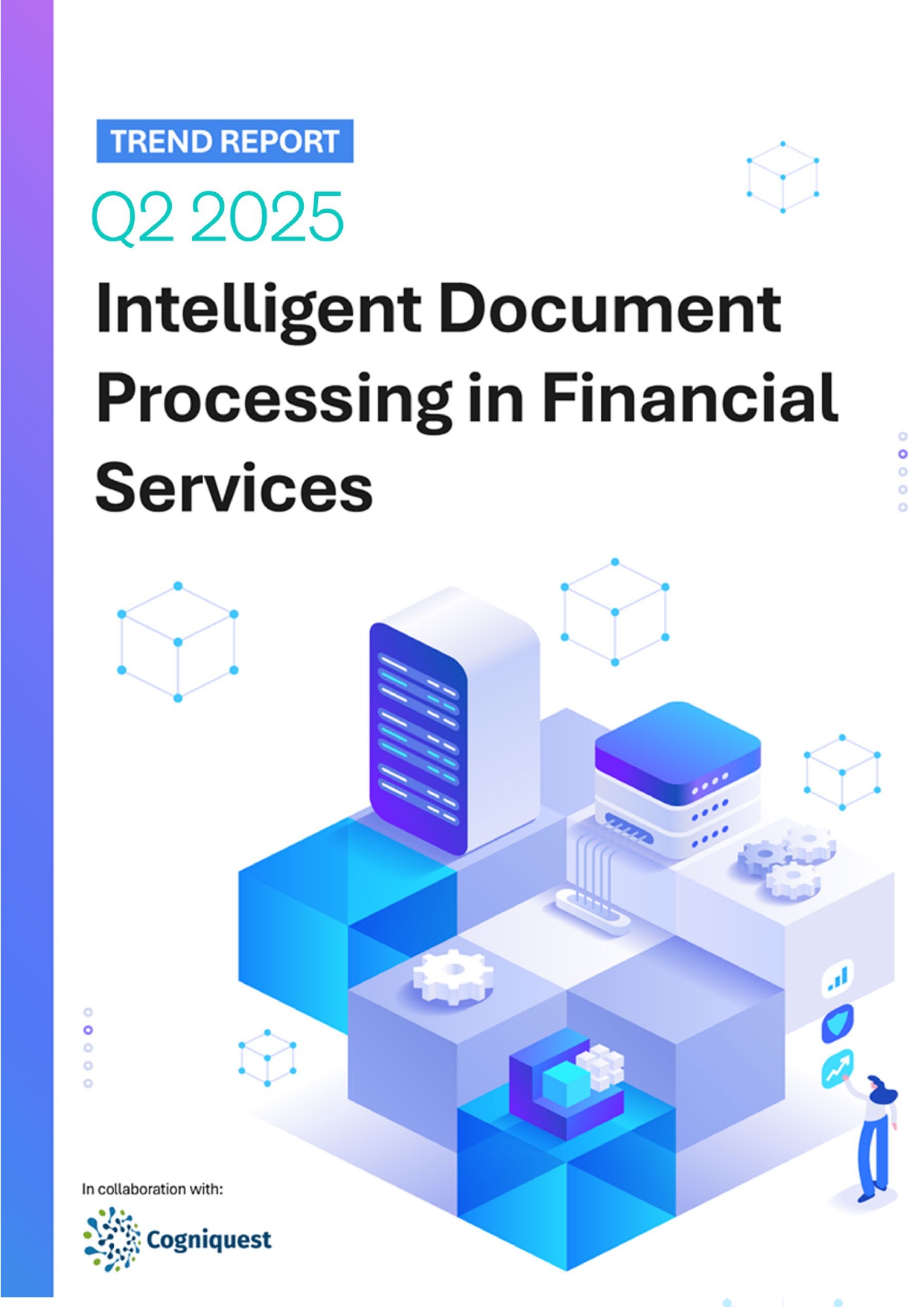
Intelligent Document Processing in Financial Services Q2 2025
Know More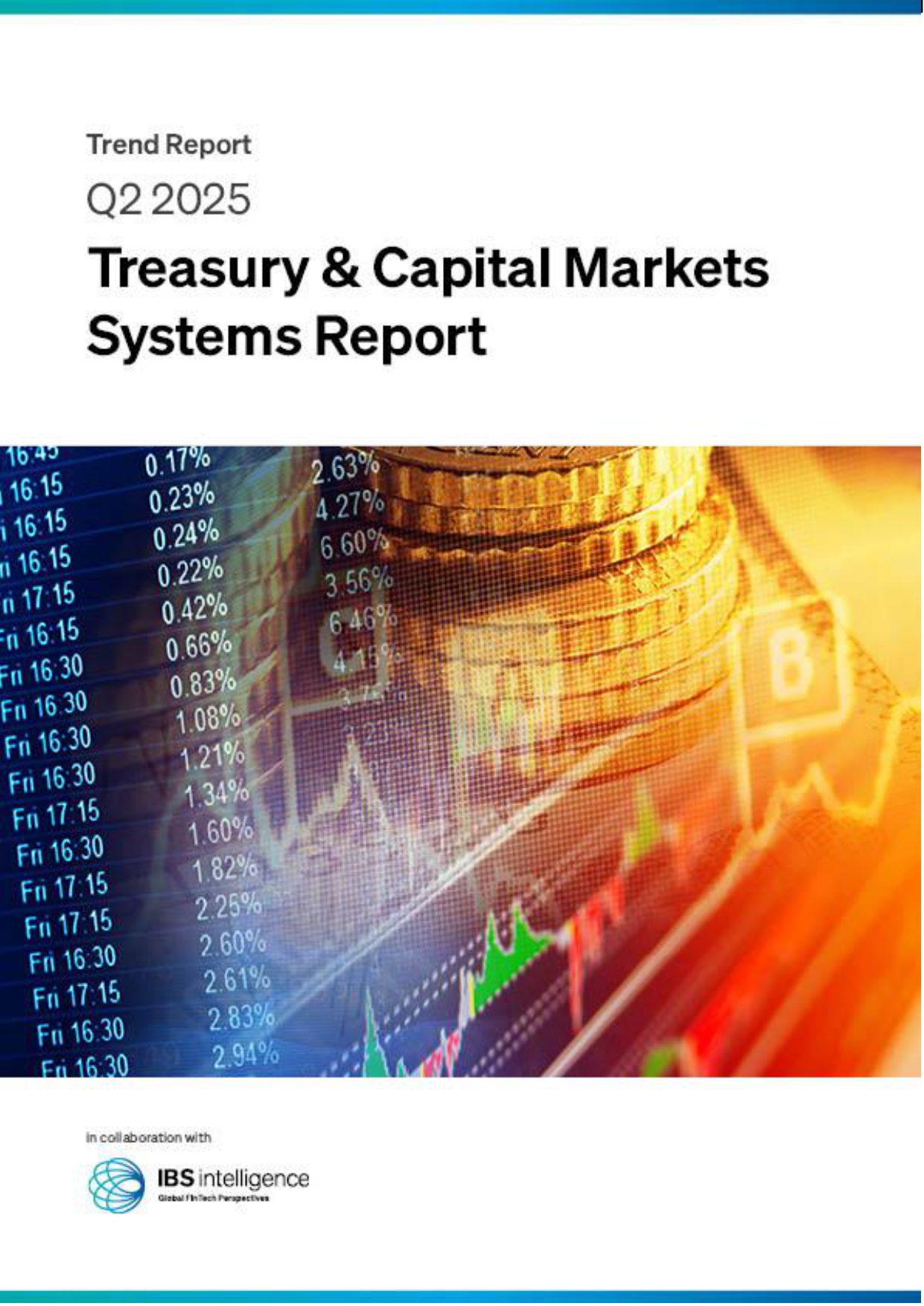